RESEARCH
RESEARCH
Gather Stakeholder Pieces
We spoke with one government official, two removal workers, and one rider to narrow down our goal with a comprehensive vision.
Municipality Clerk
“The bike removal shows low efficiency and high randomness.”
Removal Worker
“We won't usually tow bikes that are fastened to poles or trees.”
Cycler
“It's a bet; most of the time, I won't get penalized for improper parking, so I don't bother with a bike rack.”
Current Situation:
Regulate by AFAC (Bike Removal Company)

Unconfident of Bike Parking Distribution
The government cannot track clear data and trends to make bike rack iteration
Distrust for Bike Removal Company’s Progress
The extent of the government's engagement in the mutual enhancement of the bike removal company is limited.
Poor Manual Monitor Accuracy
Underreporting is typical because of the randomization of fine lists, which involve different times, different inspectors, and variable cycling conditions.
HOW CAN WE...
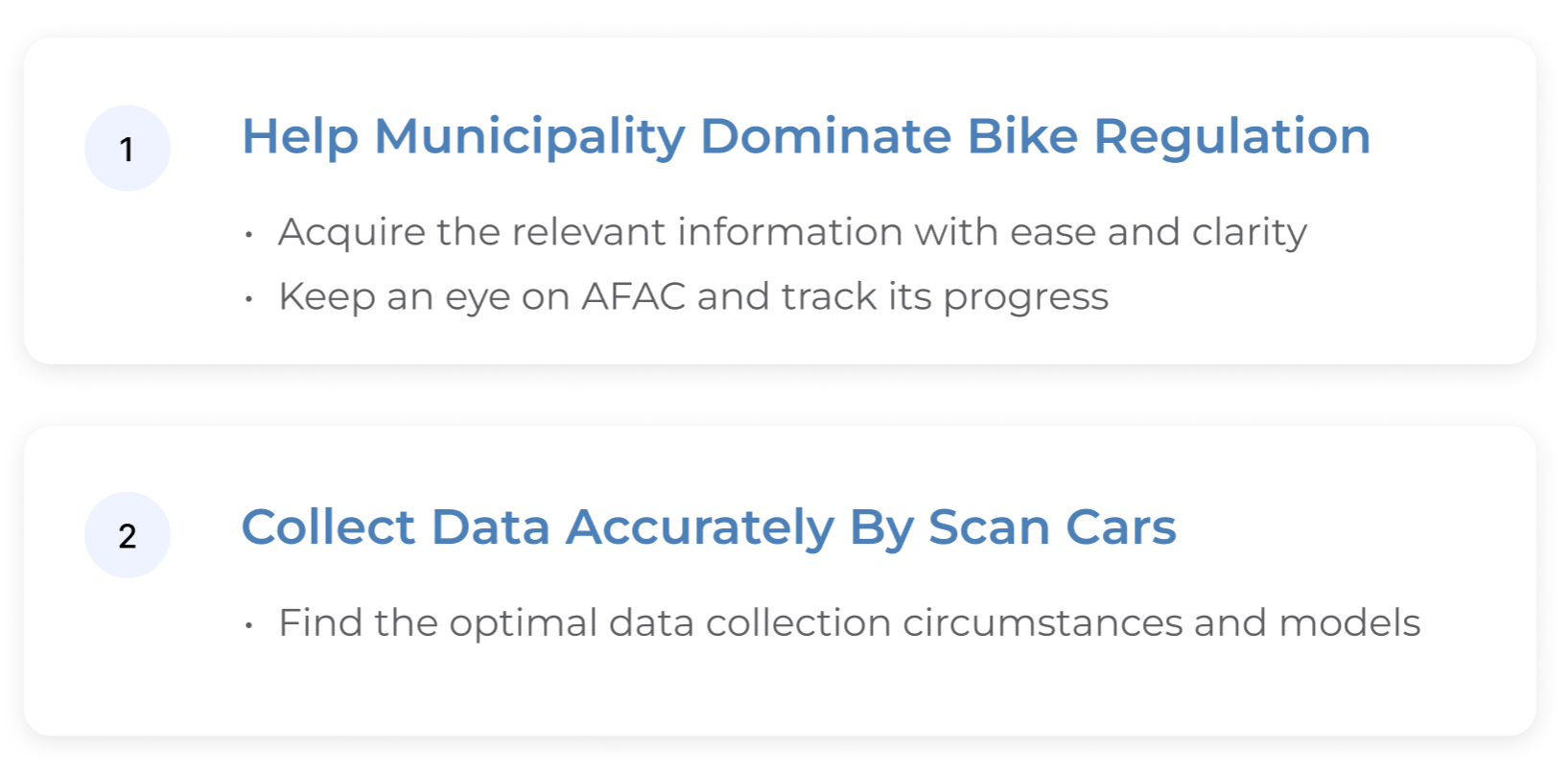